In this tutorial we will show you how to use rust-bert
library to utilize the state-of-the-art natural language processing models in Rust, and we are specifically tested on macOS environments.
Rust crate rust_bert implementation of the BERT language model (https://arxiv.org/abs/1810.04805 Devlin, Chang, Lee, Toutanova, 2018). The base model is implemented in the bert_model::BertModel struct. Several language model heads have also been implemented, including:
- Masked language model: bert_model::BertForMaskedLM
- Multiple choices: bert_model:BertForMultipleChoice
- Question answering: bert_model::BertForQuestionAnswering
- Sequence classification: bert_model::BertForSequenceClassification
- Token classification (e.g. NER, POS tagging): bert_model::BertForTokenClassification
Transformers #
Transformers is a State-of-the-art Machine Learning for Pytorch, TensorFlow, and JAX.
Before installing the transformers:
python3 -m venv .env
source .env/bin/activate
brew install cmake
brew install pkg-config
brew install sentencepiece
pip install sentencepiece
pip install transformers
pip install 'transformers[torch]'
pip install 'transformers[tf-cpu]'
pip install 'transformers[flax]'
pip install onnxruntime
Verify the installation:
(.env) ➜ transformers git:(main) python -c "from transformers import pipeline; print(pipeline('sentiment-analysis')('we love you'))"
No model was supplied, defaulted to distilbert-base-uncased-finetuned-sst-2-english and revision af0f99b (https://huggingface.co/distilbert-base-uncased-finetuned-sst-2-english).
Using a pipeline without specifying a model name and revision in production is not recommended.
Downloading (…)lve/main/config.json: 100%|████████████████████████████████████████████████████████████████████████████████████████████████| 629/629 [00:00<00:00, 1.13MB/s]
Downloading model.safetensors: 100%|████████████████████████████████████████████████████████████████████████████████████████████████████| 268M/268M [00:26<00:00, 10.0MB/s]
Downloading (…)okenizer_config.json: 100%|███████████████████████████████████████████████████████████████████████████████████████████████| 48.0/48.0 [00:00<00:00, 158kB/s]
Downloading (…)solve/main/vocab.txt: 100%|███████████████████████████████████████████████████████████████████████████████████████████████| 232k/232k [00:00<00:00, 554kB/s]
Xformers is not installed correctly. If you want to use memory_efficient_attention to accelerate training use the following command to install Xformers
pip install xformers.
[{'label': 'POSITIVE', 'score': 0.9998704195022583}]
Install the huggineface’s transformers:
git clone https://github.com/huggingface/transformers.git
cd transformers
pip install -e .
🤗 Transformers is tested on Python 3.6+, PyTorch 1.1.0+, TensorFlow 2.0+, and Flax. Follow the installation instructions below for the deep learning library you are using:
- PyTorch installation instructions.
- TensorFlow 2.0 installation instructions.
- Flax installation instructions.
So we will need to install these 3 dependend projects as follow.
Install PyTorch #
pip3 install torch torchvision torchaudio
Install TensorFlow #
# There is currently no official GPU support for MacOS.
python3 -m pip install tensorflow
# Verify install:
python3 -c "import tensorflow as tf; print(tf.reduce_sum(tf.random.normal([1000, 1000])))"
Install Flax #
Flax delivers an end-to-end and flexible user experience for researchers who use JAX with neural networks. Flax exposes the full power of JAX. It is made up of loosely coupled libraries.
JAX is a project designed for High-Performance Array Computing,
python3.11 -m pip install --upgrade pip
pip install flax
Init Rust BERT #
brew install libtorch
brew link libtorch
brew ls --verbose libtorch | grep dylib
export LIBTORCH=$(brew --cellar pytorch)/$(brew info --json pytorch | jq -r '.[0].installed[0].version')
export LD_LIBRARY_PATH=${LIBTORCH}/lib:$LD_LIBRARY_PATH
git clone https://github.com/guillaume-be/rust-bert.git
cd rust-bert
ORT_STRATEGY=system cargo run --example sentence_embeddings
Also better to add the following to bash/zsh environment in case you met exception like "libtch/torch_api_generated.cpp" with args "c++" did not execute successfully (status code exit status: 1).
:
export LIBTORCH=$(brew --cellar pytorch)/$(brew info --json pytorch | jq -r '.[0].installed[0].version')
export LD_LIBRARY_PATH=${LIBTORCH}/lib:$LD_LIBRARY_PATH
In Rust’s project #
Import the example code into Pizza’s module:
use log::*;
use rust_bert::pipelines::translation::{Language, TranslationModelBuilder};
pub(crate) fn translation() -> anyhow::Result<()> {
info!("start translation:");
let model = TranslationModelBuilder::new()
.with_source_languages(vec![Language::English])
.with_target_languages(vec![
Language::Spanish,
Language::French,
Language::Italian,
])
.create_model()
.unwrap();
let input_text = "Hello world!";
let output = model
.translate(&[input_text], None, Language::Spanish)
.unwrap();
for sentence in output {
info!("Output: {}", sentence);
}
Ok(())
}
And we will get the result as follows:
___ _____ __________ _
/ _ \\_ \/ _ / _ / /_\
/ /_)/ / /\/\// /\// / //_\\
/ ___/\/ /_ / //\/ //\/ _ \
\/ \____/ /____/____/\_/ \_/
[PIZZA] The Next-Gen Real-Time Hybrid Search & AI-Native Innovation Engine.
[2023-06-03 19:00:32] [INFO] [pizza:96] PIZZA now starting.
[2023-06-03 19:00:32] [INFO] [pizza::modules::api:71] api listen at: http://0.0.0.0:2900
[2023-06-03 19:00:32] [INFO] [pizza::modules:37] started module [api]
[2023-06-03 19:00:32] [INFO] [pizza::modules::bert::translation:4] start translation:
[2023-06-03 19:00:32] [INFO] [actix_server::builder:200] starting 8 workers
[2023-06-03 19:00:32] [INFO] [actix_server::server:197] Tokio runtime found; starting in existing Tokio runtime
[2023-06-03 19:00:33] [INFO] [cached_path::cache:414] Cached version of https://huggingface.co/Helsinki-NLP/opus-mt-en-ROMANCE/resolve/main/vocab.json is up-to-date
[2023-06-03 19:00:33] [INFO] [cached_path::cache:414] Cached version of https://huggingface.co/Helsinki-NLP/opus-mt-en-ROMANCE/resolve/main/source.spm is up-to-date
[2023-06-03 19:00:34] [INFO] [cached_path::cache:414] Cached version of https://huggingface.co/Helsinki-NLP/opus-mt-en-ROMANCE/resolve/main/config.json is up-to-date
[2023-06-03 19:00:36] [INFO] [cached_path::cache:414] Cached version of https://huggingface.co/Helsinki-NLP/opus-mt-en-ROMANCE/resolve/main/rust_model.ot is up-to-date
[2023-06-03 19:00:36] [INFO] [pizza::modules::bert::translation:21] Output: ¡Hola mundo!
[2023-06-03 19:00:36] [INFO] [pizza::modules:37] started module [bert]
[2023-06-03 19:00:36] [INFO] [pizza::modules:39] all modules are started
[2023-06-03 19:00:36] [INFO] [pizza:116] PIZZA is up and running now. PID: 37426
Wow, Hello World
was successfully translated to ¡Hola mundo!
, which is great!
As you can see, creating a translation application is incredibly simple just in a few lines of code. And this is just the beginning! By harnessing the power of these pre-trained language models, we can accomplish so much more.
Links #
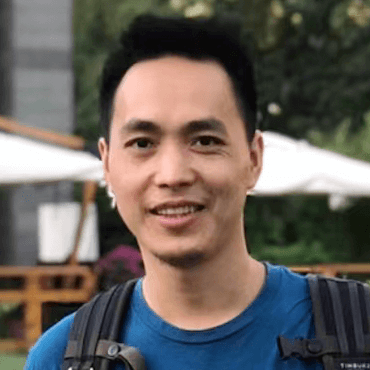